A novel A.I.-powered method developed by a team of Brown University researchers offers a fast, cost-effective way to understand protein structures in multiple dimensions, making it a useful tool for pharmacology.
PROVIDENCE, R.I. [Brown University] — Understanding the structure of proteins is critical for demystifying their functions and developing drugs that target them. To that end, a team of researchers at Brown University has developed a way of using machine learning to rapidly predict multiple protein configurations to advance understanding of protein dynamics and functions.
A study describing the approach was published in Nature Communications on Wednesday, March 27.
The authors say the technique is accurate, fast, cost-effective and has the potential to revolutionize drug discovery by uncovering many more targets for new treatments.
In targeted cancer therapy, for example, treatments are designed to zero in on proteins that control how cancer cells grow, divide and spread. One of the challenges for structural biologists has been understanding cell proteins thoroughly enough to identify targets, said study author Gabriel Monteiro da Silva, a Ph.D. candidate in molecular biology, cell biology and biochemistry at Brown.
Monteiro da Silva uses computational methods to model protein dynamics and looks for ways to improve methods or find new methods that work best for different situations. For this study, he partnered with Brenda Rubenstein, an associate professor of chemistry and physics, and other Brown researchers to experiment with an existing A.I.-powered computational method called AlphaFold2.
While Monteiro da Silva said that the accuracy of AlphaFold2 has revolutionized protein structure prediction, the method has limitations: It allows scientists to model proteins only in a static state at a specific point in time.
“During most cellular processes, proteins will change shape dynamically,” Monteiro da Silva said. “In order to match protein targets to drugs to treat cancer and other diseases, we need a more accurate understanding of these physiological changes. We need to go beyond 3D shapes to understanding 4D shapes, with the fourth dimension being time. That’s what we did with this approach.”
Monteiro da Silva used the analogy of a horse to explain protein models. The arrangement of the horse’s muscles and limbs create different shapes depending on whether the horse is standing or galloping; protein molecules conform into different shapes due to the bonding arrangements of their constituent atoms. Imagine that the protein is a horse, Monteiro da Silva said. Previous methods were used to predict a model of a standing horse. It was accurate, but it didn’t tell much about how the horse behaved or how it looked when it wasn’t standing.
In this study, the researchers were able to manipulate the evolutionary signals from the protein to use AlphaFold2 to rapidly predict multiple protein conformations, as well as how often those structures are populated. Using the horse analogy, the new method allows researchers to quickly predict multiple snapshots of a horse galloping, which means they can see how the muscular structure of the horse would change as it moved, and then compare those structural differences.
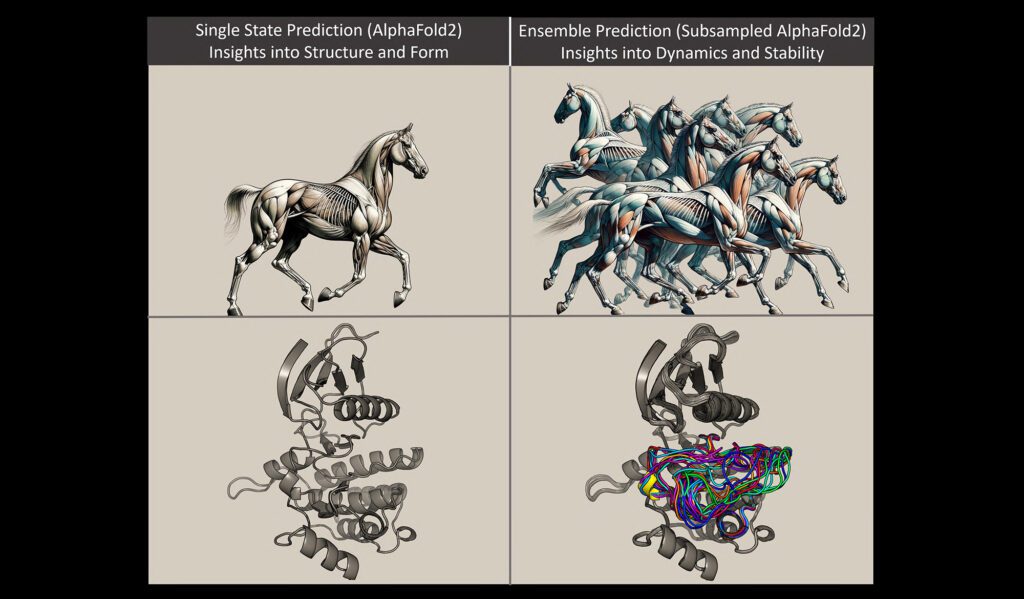
“If you understand the multiple snapshots that make up the dynamics of what’s going on with the protein, then you can find multiple different ways of targeting the proteins with drugs and treating diseases,” said Rubenstein, whose research focuses whose research focuses on electronic structure and biophysics.
Rubenstein explained that the protein on which the team focused in this study was one that had different drugs developed for it. Yet for many years, no one could understand why some of the drugs succeeded or failed, she said.
“It all came down to the fact that these specific proteins have multiple conformations, as well as to understanding how the drugs bind to the different conformations, instead of to the one static structure that these techniques previously predicted; knowing the set of conformations was incredibly important to understanding how these drugs actually functioned in the body,” Rubenstein said.
Accelerating discovery time
The researchers noted that existing computational methods are cost- and time-intensive.
“They’re expensive in terms of materials, in terms of infrastructure; they take a lot of time, and you can’t really do these computations in a high throughput kind of way — I’m sure I was one of the top users of GPUs in Brown’s computer cluster,” Monteiro da Silva said. “On a larger scale, this is a problem because there’s a lot to explore in the protein world: how protein dynamics and structure are involved in poorly understood diseases, in drug resistance and in emerging pathogens.”
The researchers described how Monteiro da Silva previously spent three years using physics to understand protein dynamics and conformations. Using their new A.I.-powered approach, the discovery time decreased to mere hours.
“So you can imagine what a difference that would make in a person’s life: three years versus three hours,” Rubenstein said. “And that’s why it was very important that the method we developed should be high-throughput and highly efficient.”
As for next steps, the research team is refining their machine learning approach, making it more accurate as well as generalizable, and more useful for a range of applications.
The study was supported by the Blavatnik Family Foundation, which funds a graduate fellowship in biology and medicine at Brown University. Eight Blavatnik Family Fellows were selected in Fall 2023 based on outstanding academic achievement and demonstrated potential for producing research that advances scientific knowledge and understanding in the basic and clinical life sciences. Monteiro da Silva is one of the inaugural fellows, as is co-author Jennifer Cui, who is analyzing the structure and function of proteins involved in inflammation and cell signaling with fellow co-author George Lisi, a professor of molecular biology, cell biology and biochemistry.
MEDIA CONTACT
Corrie Pikul
corrie_pikul@brown.edu
401-863-1862